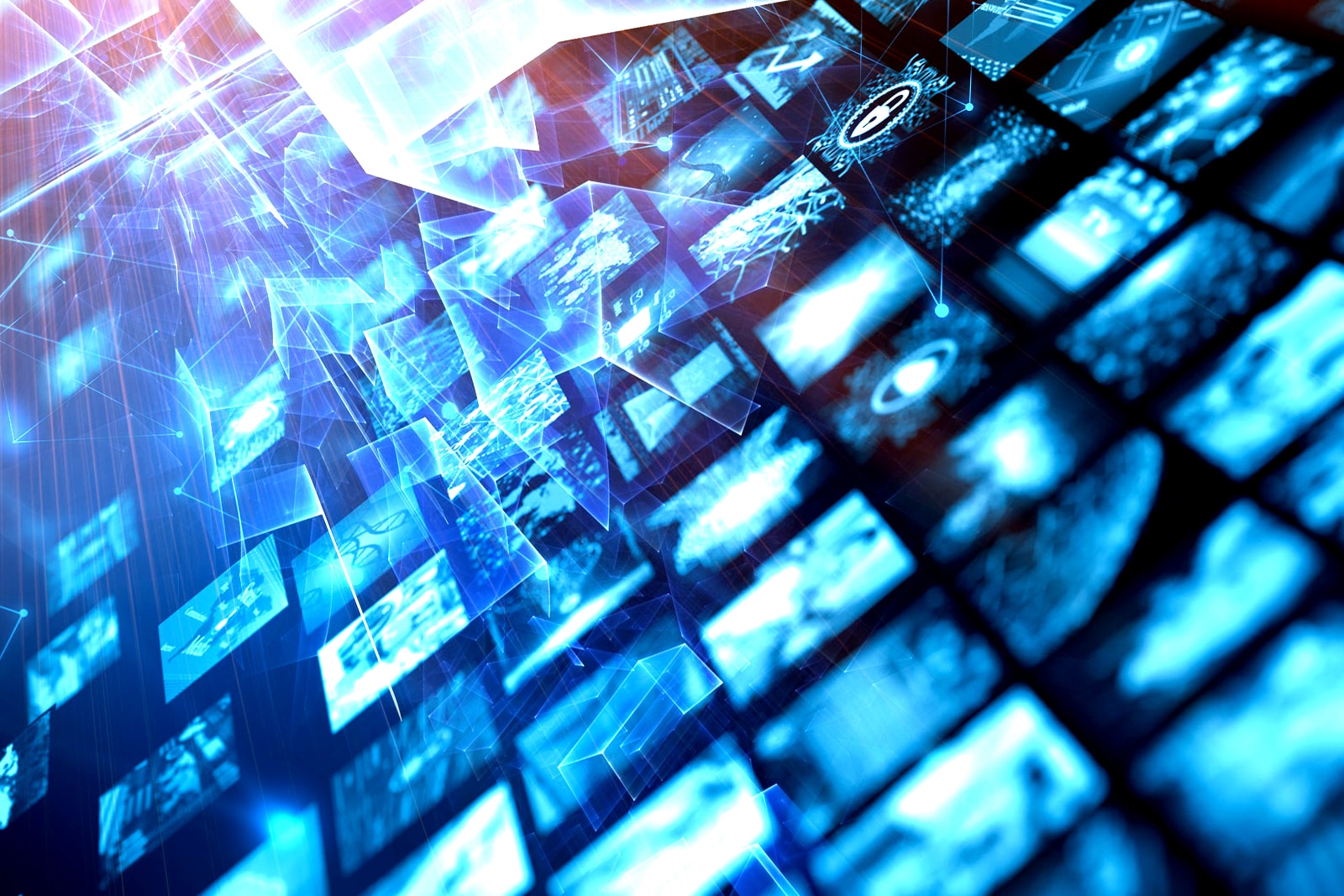
Becoming a data scientist has its perks, but it also comes with its own set of unique challenges. For example, you can spend hours or days trying to make sense of a data set only to find out that it has been totally mislabeled, corrupted, or just completely useless because of an upstream problem (that’s beyond your control).
Data scientists on occasion are also asked to analyze data derived from poorly designed and implemented tests or experiments. This, in turn, can be frustrating as the data that has already been collected can be pure garbage.
But what can be considered the worst nightmare for a data scientist? I’ve figured at least 3, let’s take a look.
1. Making a mistake that affects the whole strategy
We have all been guilty of making mistakes on the job at one time or another, but a mistake made by a data scientist can have far reaching consequences. In fact, this is the worst nightmare of SafeGraph’s data scientist, Ryan Fox Squire.
Here’s what he had to say about it!
“I've discovered something important and unexpected in our data. I double check it. I triple check it. I share it with my teammates and they give me some interesting feedback and follow up points. All the follow ups check out. We share it more broadly—it's important and people listen to me, so it starts affecting strategy. Business and product decisions start getting changed based on my analysis. Resources are invested. Opportunity costs are lost. Then I realize I made a mistake, and it's all wrong.”
It’s something that keeps him up at night and I am sure that there will be thousands more who can relate to this scenario. At the end of the day, data science is essentially a quantitative field, so the value you bring to the job will significantly depend on the trust of your colleagues.
Trust is key as your colleagues won’t be able to review all your code or even understand the models that were used. So making sure that it’s all correct comes down solely to you.
2. Clients that question the quality of data discovery
Sometimes the problem isn’t data at all, instead, it’s a human one. Think about it, you comb through the data, develop models, and derive some real business value from good data, but the client doesn’t want to accept your findings.
A lot of times, companies are guilty of historically doing the same thing, so when you come in with your findings, it can be a bit much to swallow. When they find out that what they have done over and over again for many years was ineffective, you can quickly become the enemy even though your findings are totally right.
In this scenario, you can’t really blame the client as they might also have serious consequences for admitting and accepting that mistakes were made (they might even lose their job!). Then there can also be times when your findings are used inappropriately attack another colleague.
Check out how to distinguish a real data scientist from a faker.
3. Cleaning data
While the above is known to happen, most often the common nightmare among most data scientists is messy and unstructured data. No matter what industry you’re working in, this is something that is more or less guaranteed to happen.
Whether it’s badly planned, implemented, or poorly designed, cleaning up the data is an important part of the job. But it also can be the most tedious and frustrating part of one’s career in data science.
And what keeps you up at night?