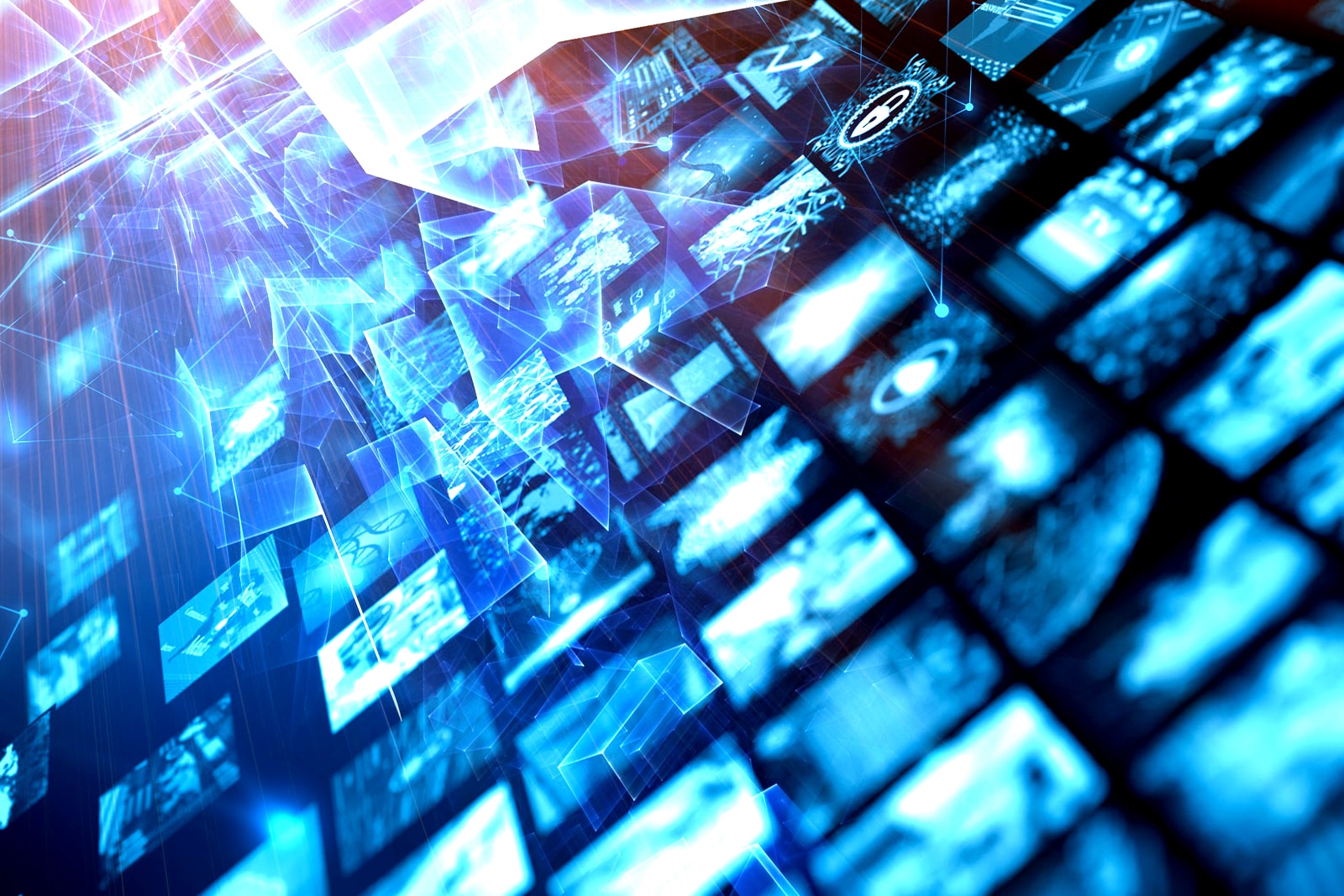
In the digital age, you hear a lot about data scientists and data analysts. But who should you hire for your big data project? While there is significant overlap between the two roles, there are also major differences.
This means that you have to first figure out what your organization is trying to achieve by taking advantage of big data.
Data Analyst vs Data Scientist
A data analyst usually works in a junior role and does the groundwork for the data scientist. They usually run queries against new data to identify new trends, produce intuitive visuals, and write scripts.
These individuals are usually SQL experts who help prepare the data for data scientists.
A data scientist is responsible for building models utilizing machine learning (ML). These models are typically expected to engender the company’s software with adaptive product features that are able to analyze and make predictions.
The quality of the models they build will depend completely on how well the data is prepared and understood. This is the primary reason why data analysts and scientists work closely together to understand and prepare the data to build better models.
While data scientists and data analysts do have a lot in common, both jobs are definitely different. For example, data scientists are expected to possess strong business acumen and data visualization skills. It’s important as they are tasked with turning insights into business stories.
A data analyst isn’t expected to have any business acumen or advanced data visualization skills. Furthermore, data analysts just look at the data available on a single source whereas data scientists explore and examine data from a variety of disconnected sources.
The data scientist is the one who will formulate the issue whose solutions will most probably benefit the company. The data analyst, on the other hand, will find an answer to the question provided by the business.
But the biggest difference between the two roles is the fact that data analysts aren’t expected to be Machine Learning (ML) experts. Additionally, they are also not expected to build statistical models.
Data analysts also often find themselves in more client facing roles where the tasks are directly related to tangible business needs. Data scientists are usually more free as they are not trying to find an answer to a customer’s question. Instead, they usually look through different data sets to try and formulate a question.
So if your company doesn’t know how to take advantage of big data or understand how the available data can benefit the business, then it’s probably best to hire a data scientist.
There is also another related role that you don’t hear about often, but it’s still important. Data engineers are the individuals tasked with taking what was developed and putting it into production. They work closely with data scientists to ensure that they place the ML models correctly.
Sometimes, data engineers also work with data analysts to make sure that data ingestion and conversion is taken into account from the right sources. But all three play a critical role in converting raw data to deployed features inside products.
Featured image courtesy of Digi117